Meta's Multi-Token Model: A Leap Forward in AI
Meta has introduced a groundbreaking approach to training large language models (LLMs) called multi-token prediction. By predicting multiple tokens simultaneously, instead of one at a time, this method significantly boosts model speed and accuracy, particularly in code generation tasks.
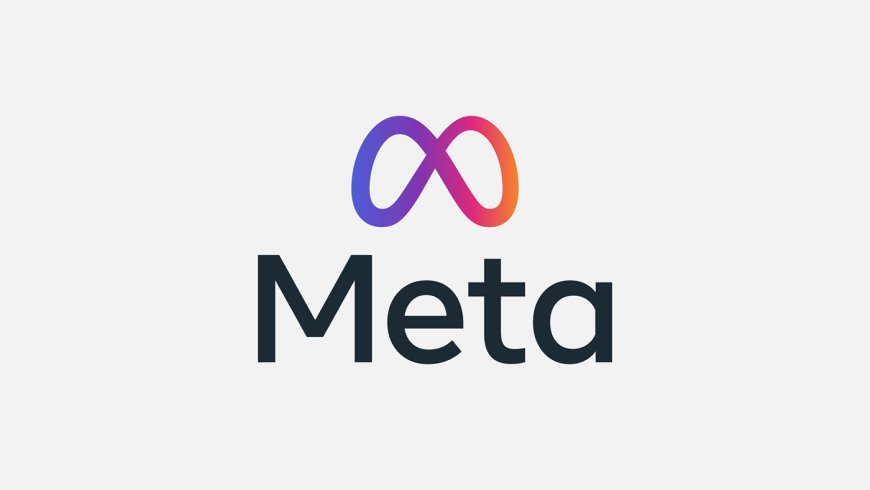
The landscape of artificial intelligence is constantly evolving, with new breakthroughs emerging at an unprecedented pace. One such innovation is Meta's multi-token prediction model, a departure from the traditional next-token prediction paradigm used in large language models (LLMs).
Unlike conventional LLMs that predict one token at a time, Meta's model is designed to forecast multiple tokens simultaneously. This novel approach brings several advantages to the table:
- Enhanced Speed: Multi-token prediction models can process information up to three times faster than their predecessors, making them highly efficient for real-world applications.
- Improved Accuracy: These models have demonstrated superior performance on various benchmarks, especially in the realm of code generation.
- Deeper Understanding: By predicting multiple tokens at once, the model gains a better grasp of long-range dependencies within the text, leading to more coherent and contextually relevant outputs.
Meta's commitment to open-source development has seen the release of four multi-token prediction models, each equipped with 7 billion parameters. These models have been trained on extensive datasets and exhibit exceptional capabilities in code generation tasks.
The implications of this technology are far-reaching. From accelerating software development to enhancing natural language processing, multi-token prediction has the potential to revolutionize multiple industries. As research progresses, we can anticipate even more sophisticated and powerful models emerging in the near future.